Scanning our hearts
We scanned our hearts and incidentally found them full of love and appreciation for our readers – you’re all our valentines!
The love story begins…
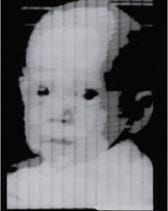
"What would happen if computers could look at pictures?"
In 1957 – the first digital image was created by Russell Kirsch – ushering in a new field of multi-modality computer applications. Within another 20 years Godfrey Hounsfield developed the first clinical CT scanner and is soon followed up by the first completed MRI by Raymond Damadian in 1977. These imaging technologies are a far cry from where we are today, in fact, in this error, these scans were still being read on a light-board. It wasn't until the mid-1980's that the American College of Radiology (ACR) and National Electrical Manufacturers Association (NEMA) partnered on an initiative to foster a standard for medical imaging storage and transmission. This project would culminate in the Digitial Imaging and Communications in Medicine (DICOM) standard (which, by the way, is still the gold standard to this day) underpinning the technology that would bring to life the error of Picture Archiving and Communication Systems (PACS) and breathe life into the digital radiology industry that is thriving to this day. Because of this early push toward digital, Radiology has always been leading the pack of technology adoption in healthcare. Knowing this, it’s likely obvious to hear that the industry’s advocacy for implementing Artificial Intelligence (AI) is likely the highest of all healthcare fields. But how? How is AI used in radiology? Does AI read all our cases now? Not quite.
Let’s follow our patient – Johnny – on his visit to the Emergency Dept for a hard fall in a town softball game. We’ll see how the industry is injecting AI to help increase efficiency, respond quicker and ultimately allow our providers to be more human again.
Image Optimization
Upon initial examination in the Emergency Department, Johnny is sent for a CT of his head and neck to rule out any internal bleeding caused by the trauma to his head when he tripped over first base hustling out a hard ground ball.
Without AI
Johnny is brought back to the CT scanner, is laid down on the table and the technologists starts to position the patient appropriately. This generally consists of the patient lying on the table and the technologist, with the help of a positioning light, moving the patient on the table and the patient performing any final tweaks to his/her positioning to line the scan up just right. The technologist will then take a scout image and confirm positioning, making any last-minute adjustments before commencing the full scan. With experienced technologists at the helm and cooperative patients on the table, this is normally a relatively straightforward task, normally only requiring 1 or 2 re-positioning cycles.
Total Time: 40 mins
With AI
Johnny is brought back to the CT scanner, is laid down on the table and the technologist starts to position the patient appropriately. Thanks to companies like GE and Siemens the room is equipped with an auto-positioning system that leverages a top down 2D/3D camera to position the patient optimally based on size, body composition and reference markers automatically calculated on the device. Using this system the technologists have the patient positioned well. They confirm their scout and commence the final scan.
Total Time: 30 mins
Image Reconstruction
While Johnny is assisted back to his bed, his images travel to their next destination.
Without AI
These images are sent over to the PACS (picture archiving and communication system) and the exam is thrown into the queue for reading. No priority is given to the case, and the raw images are the entirety of what the radiologist is given to read. This all happens automatically and in short order (within a few minutes), but the case is now one of 50 studies to be read by the team of three radiologists working off of a worklist.
Total Time: 10 mins
With AI
These images are sent over to the PACS and the exam is thrown into the queue for reading. Simultaneously, the thin slices (taken during the original exam) are sent over to a TeraRecon post-processing workstation. There, AI models build a 3D representation of the anatomy out of the static images. These images are also sent to the PACS. No priority is given to the case; however the radiologist now has a 3D reconstruction alongside the raw imaging to help inform their report. This all happens automatically and in short order (within a few minutes), but the case is now one of 50 studies to be read by the team of three radiologists working off of a worklist.
Total Time: 10 mins
Note: We mention TeraRecon, but there are other companies in this space such as Tempus, Qure.ai, Viz.AI
Detection of abnormalities and case prioritization
After Johnny gets back to his bed, he waits around anxiously as his case slowly moves up the worklist for the radiologist to read.
Without AI
Given there was no priority put on Johnny’s case it is lumped in with the rest of the cases from the ED and simply needs to wait its turn.
Total Time: 60 mins
With AI
While the thin slices are being reconstructed, the entire study is also sent over to Aidoc’s aiOS, where it is run through a series of computer vision models searching for abnormalities in the scan. One of the models reports a suspicious finding in the case. The system sends a notification to the worklist system, increasing the priority of the case, automatically elevating it on the radiologist’s worklist. Because of this priority, the case is bumped to the top of the worklist and the radiologist starts to read the case with urgency.
Total Time: 20 mins
Note: We mention AiDoc in this instance but there are many companies in this space, such as Viz.ai, Rapid, Nanox, and Qure.ai, to name a few, all of which have FDA-cleared solutions in this space.
Report writing
The radiologist gets to Johnny’s case and begins his interpretation of the exam.
Without AI
The provider notices an abnormality in the scan but considers it non-urgent. He starts typing to capture the findings and impression of the case. He then signs Johnny’s report and moves on to the next case. As you can imagine, his fingers are more than tired by the end of his shift and the quality of his typing decreases steadily throughout the night.
Total Time: 30 mins
With AI
Because of the health system’s AI-forward strategy, they have integrated Rad AI’s tools, allowing the software to take a first pass on the report given the images, prior reports, and encounter context. The radiologist opens the case and immediately notices the abnormality that the algorithm correctly pointed out in the scan but considers it non-urgent. He moves over to the report, corrects a few sentences in the auto-created report draft created by Nuance PowerScribe 360’s speech to text software, ensuring coverage of all findings and ensures the impression is accurate and of correct format and tone. He then signs Johnny’s report and moves on to the next case. Because the radiologist is able to complete this reporting workflow through dictation instead of typing, he has avoided his carpal tunnel fate, and the quality of his dictated report remains constant throughout his shift. His voice profile is always adapting alongside his personalized reporting style used by the Rad AI workflow, so over time the quality of the dictation will also improve, leading to less corrections.
Total Time: 10 mins
Check for accuracy
Without AI
The radiologist inadvertently included the wrong sex of Johnny in his report, causing it to fail to post upstream and the ordering physician to not be notified of its completion. This failure alerted an IT member on-call to fix the issue by sending the report back to the radiologist to amend. This process is burdensome for everyone involved and adds a considerable amount of time to the case.
Total Time: 75 mins
With AI
Once the radiologist attempts to sign off on the exam, Nuance fires off its Quality Check algorithm that scans the report (given the patient's history, previous reports, demographics, reason for exam, etc.) for inconsistencies in the report text. It finds the sex mismatch, highlights the offending text and the radiologist corrects it live. Once all corrections are made, the report is successfully sent out and posts in downstream systems, alerting the ordering physician of the report completion.
Total Time: 5 mins
Patient communication
Without AI
Since the scan returned a clean bill of health and the finding was unimpressionable, the attending physician sends Johnny home to be with Moira. He gives Johnny a concussion protocol and instructs home to try a little less hard at the neighborhood softball games – reminding him that his shot at the big leagues has long passed. The attending does not mention the incidental finding on Johnny’s report. Johnny is discharged and he does not read his report, nor does he ever schedule a follow-up for the lump found in his neck.
Total Time: 60 mins
With AI
Johnny, while waiting for the attending to enter his room and give him the good news, gets an alert on his phone. The EMR app that he has installed alerts him of the finalized radiology report and sends him a link to view it. The link sends Johnny over to a report created by Scanslated, which allows Johnny to actively understand the contents of the radiologist’s report. When the attending physician enters the room, he and Johnny have an informed conversation about the results, which include an incidental finding in his neck. Johnny schedules a follow up right from his phone to investigate further. The attending physician sends Johnny home to be with Moira. He gives Johnny a concussion protocol and instructs home to try a little less hard at the neighborhood softball games – reminding him that his shot at the big leagues is long past. Johnny is discharged from the ED.
Total Time: 60 mins
AI helps fix radiology’s broken heart [image analysis and process automation]
Luckily for Johnny, the lump in his neck turned out to be nothing of concern and he went on to live a long happy life as the mayor a wonderful little town. As you read through this story, it should have been obvious that the AI assisted workflow was orders of magnitude better for all involved. In fact, we saw a time saving of 140 minutes (a 51% reduction in time) with the AI assisted workflow, providing a more efficient experience for the patient. The assistance from AI also helped the radiologist be more efficient with his workload, kept the on-call IT guy with his family instead of fixing a silly mistake, and helped the attending physician have an informed discussion with the patient about a potential follow-up visit, ultimately leading to additional revenue for the health system and a happy patient. You should have also noticed that at no steps in this AI-assisted radiology workflow was an AI-augmented application ever given the sole responsibility of final delivery of a task. At each of these steps there remains a human in the loop, with the application there to assist. This symbiosis brings to light what is truly possible when we allow AI to assist in a healthcare workflow.
Cost of the relationship
Venture investors have been pouring money into radiology-related companies for several years – it is perhaps one of the earliest “hot” healthtech categories. The oldest companies in the space are imaging clinical decision support software businesses that use technology to read medical imaging and interpret results for a radiologist to review. For example, Aidoc has created clinical AI tools to streamline workflows and capture clinical findings. The company has raised over $280M and has developed 15+ FDA approved algorithms across unique radiological use cases. Nanox has an AI product to read CT scans for any clinical indication, acquired through Zebra Medical Vision in 2021 for $200M. Their product specifically aims to find undiagnosed chronic conditions. Qure.ai is an India-based AI radiology company that has raised over $150M and has developed products for TB, lung cancer, and stroke that are sold globally and in use in over 90 countries. Viz.ai is another company that has raised nearly $300M and has a radiology platform that includes specialized AI tools for cardiac, neurological, and vascular applications. RapidAI (raised $100M+) has created brain imaging analysis software tools to identify and diagnose cerebrovascular disorders. TeraRecon, acquired by ConcertAI in 2021, is an imaging visualization and interpretation platform that has tools to streamline physician workflows. Tempus, a publicly traded healthcare data company (IPO in June 2024 at a $6B valuation), has created automated radiology image analysis and reporting tools.
Other radiology AI companies are focused on administrative tasks associated with imaging and communicating with patients. Companies like Rad AI are helping address radiologist burnout with workflow and follow-up management tools that automate reporting, impressions, and communication with patients to ensure care management and continuity. A company we mentioned earlier – Scanslated – converts complex clinical terms in radiology reports into easy-to-understand summaries for patients.
Finally, in the spirit of Valentine’s Day, the last company we want to mention uses AI to help providers look at hearts. HeartFlow’s non-invasive cardiac test uses AI to create 3D models of patients' coronary arteries from CT scans to help physicians create more effective treatment plans for patients. The company has raised over $800M to date and is preparing to go public.
Radiology and AI: Enemies to Lovers?
As you can see, perhaps the most ubiquitous medical AI use case is radiology. We’ve been seeing solutions roll out in this space over the last decade, as it tends to lend itself best to such products, both from a technological implementation and regulatory standpoint. Considering the recent uptick in AI-enabled solutions and Generative AI models becoming household names, it begs the question of how radiology, despite historically being ahead of the game, is going to keep up and spread the love.
Since 1995, the FDA has approved over 950 AI / ML-enabled medical devices, with a significant increase in submissions and approvals in recent years. Of those devices, over three-quarters are specific to radiology (Cupid’s arrow struck, and AI + Radiology are a match!). These solutions can range from improving quality of images, analyzing reports and scans for abnormalities, and workflow prioritization for radiologists. Topically, next on the list in terms of prevalence are cardiovascular AI solutions, though there’s a HEARTy gap between cardiovascular and radiology approval volumes.
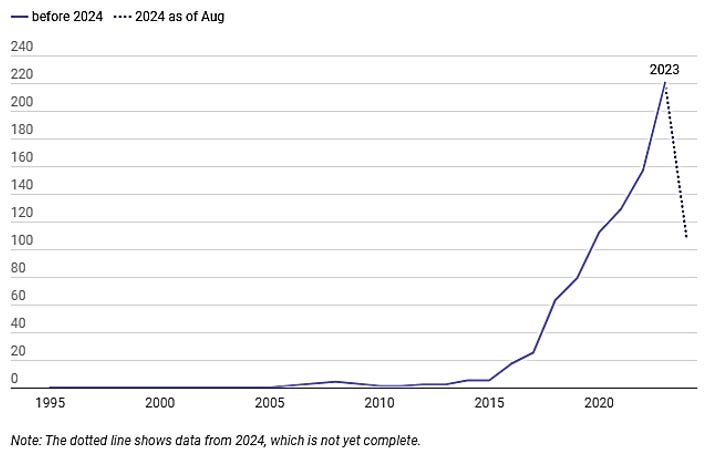
Radiology, in many ways, may very well act as the gateway for AI in new clinical and therapeutic areas. For so long, the focus had been on a constant will they, won’t they in terms of implementing AI without stepping on radiologist toes. In the meantime, it has become apparent that they will, and AI is becoming less of a threat on the dance floor and more of a partner. The emphasis on clinician + AI to fuel improved patient care is without a doubt a function of the last decade of AI courtship within radiology. Whether from the perspective of predicates enabling more efficient 510(k) approvals, proof of concepts for image-based AI, or health system infrastructure being built to accommodate data-driven AI / ML platform integration, the pathway for AI implementation across other specialties has actually benefited from the trials, tribulations, and triumphs of radiological use cases. Like any good relationship, though it started from a place of skepticism, the use of AI solutions, incorporated into the radiologist workflow, has helped build trust and increase adoption of AI, with radiologists often the entry point for a health system. In fact, as we see greater emphasis on multi-agent frameworks and multimodal data elements, AI tools may be deployed at a larger scale, incorporating radiology findings with other longitudinal patient data that involves additional therapeutic areas and diversity in data formats. While this does not mean that concerns have dissipated around the black box nature of more complex models and how best to create clinical decision support solutions that incorporate clinician-in-the-loop at every stage, it does suggest broader use cases for AI across healthcare.
Concluding Thoughts
Roses are red,
Violets are blue,
You can’t spell radiology without AI;
Come to think of it, valentines too!